“Data is a tool for enhancing intuition.”
–Hilary Mason, Data Scientist
Learning to interpret data effectively was one of my first lessons as a teacher. I came into an established classroom in December when the existing teacher took a new role. As such, I inherited a gradebook already filled with scores for the 120 students that I was now responsible for.
Since I saw the data before I met the students, I poured over those grades, making notes of which students seemed to need extra support on certain concepts and which ones may need extra challenges. I was allowed access to the records room, where I searched through folders to find years of standardized test results.
Carefully cross-referencing all of these numbers against the state math standards, I came up with a plan of action for the classes as a whole and for certain students individually.
And then once I actually met the students, all those plans were thrown out the metaphorical window. Because the quantitative data that I had only gave a piece of the picture and I had been acting as through it was the entire truth.
Quantitative data alone tends to oversimplify a situation, reducing individuals to aggregates and trends.
In some ways, what I observed validated the quantitative data. Yes, student A did struggle with integers and needed extra support. But after getting to know that student, what they really needed was some belief in themselves that they could do math. They struggled with integers because they had shut down years earlier out of a fear of being seen as stupid. The assessments measured the outcome, but didn’t reveal the underlying cause.
And student B? The one who made a 90+ on every assessment? Well, they happened to be quite skilled at applying algorithms, but nothing had yet revealed their inability to navigate a novel problem. The assessments failed to ask the right questions, so the data were incomplete. The student didn’t need harder material, they needed different material.
The early data were not wrong, but following it blindly without consideration for qualitative factors would mean that the solutions wouldn’t address – or fix – the actual problem.
It can be tempting to put too much faith in pure quantitative data. After all, since it has to obey certain rules, it seems truthier than other types of information. It’s often relatively easy to parse and hard and fast rules can be written for decisions that arise from the data. There’s a comfort in the quantitative; by reducing things, we can feel like we can control our environments. But much like a model that is too simple fails to be useful, purely quantitative data can easily become meaningless if it fails to address the underlying “why?” behind a process.
Of course, it is also possible to err on the other side, to fall into the trap of only relying on qualitative data without taking any measurements. Without the quantitative, we cannot answer the questions of “By how much?” or “Is the difference significant?”
By working with student A on building their confidence and then revisiting integer concepts, I can have a sense that they have improved, but only an assessment (or, ideally, a series of different kinds of assessment) can show the progress and put it in a form that can be communicated, analyzed and tracked over time.
Qualitative data alone tends to over-emphasize certain factors and completely ignore others.
Qualitative data is very sensitive to bias and often difficult to summarize in a meaningful and useful way. Furthermore, the sample size for qualitative data tends to be much smaller (I can get the dollar amount that 10,000 customers spent with a company a whole lot easier than I can interview each one for their feelings about the company). And when that sample size approaches 1, data are replaced with anecdotes – maybe still useful, but not actionable on their own.
To be the most informative, we need to consider both types of data together. For example, think about a winter weather forecast – the actual temperature of 35o tells you that there shouldn’t be ice on the roads (good to know!) but the “feels like” forecast of 29o warns you to wear your heavier coat. Both data points convey information and, when taken together, give you a better understanding than one alone.
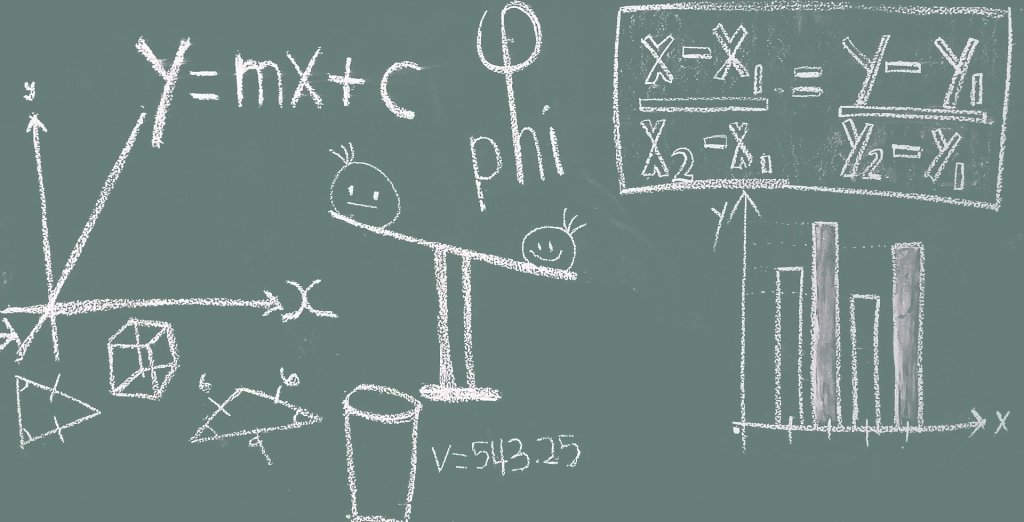
Lesson of the Day
I’ve been working my way through some business analytics courses and I learned about the three most important (at least according to this resource) customer summary metrics: recency, frequency and monetary value.
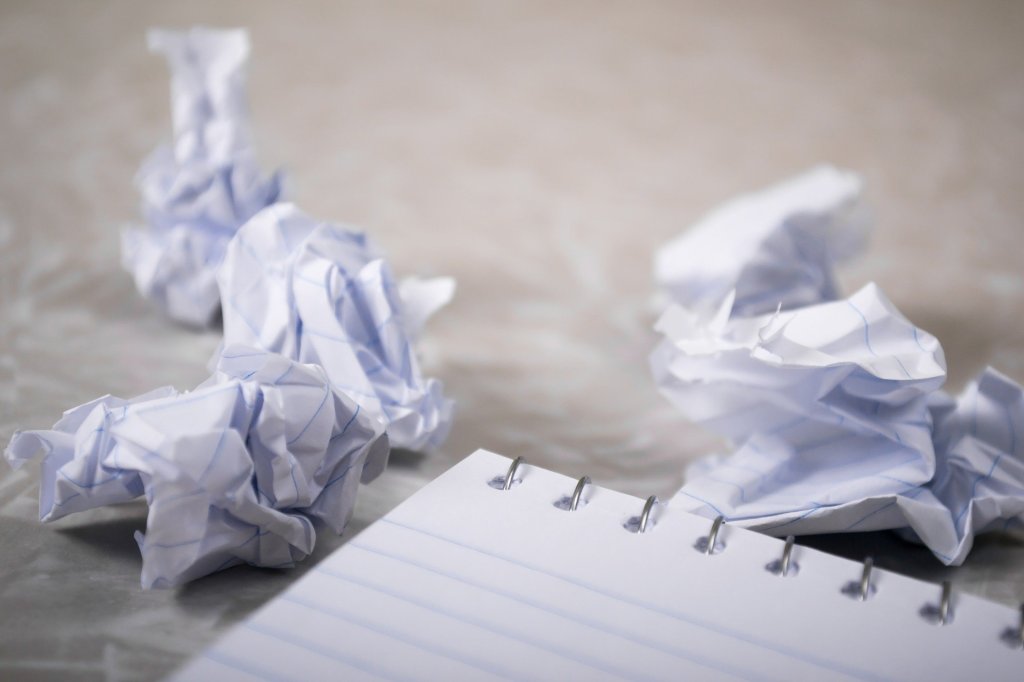
Frustration of the Day
I’m not doing well at the moment with the waiting aspect of job hunting. Patience isn’t my strongest suit.
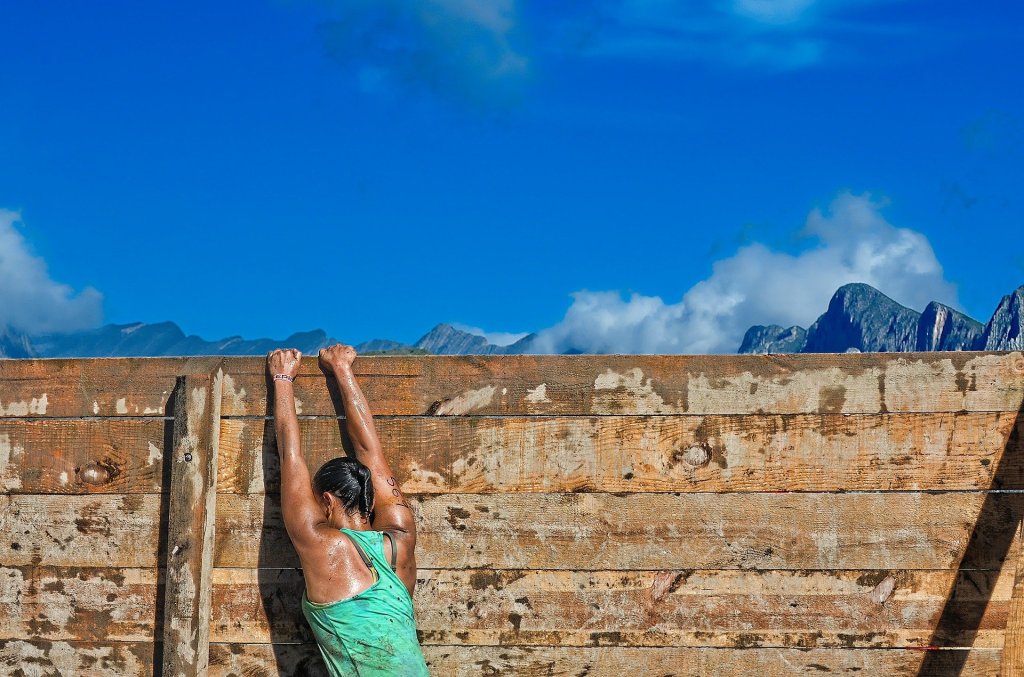
Win of the Day
I unfollowed the “leaving teaching” groups that I was a part of on Facebook. I’ve outgrown them now. I’m looking forward.
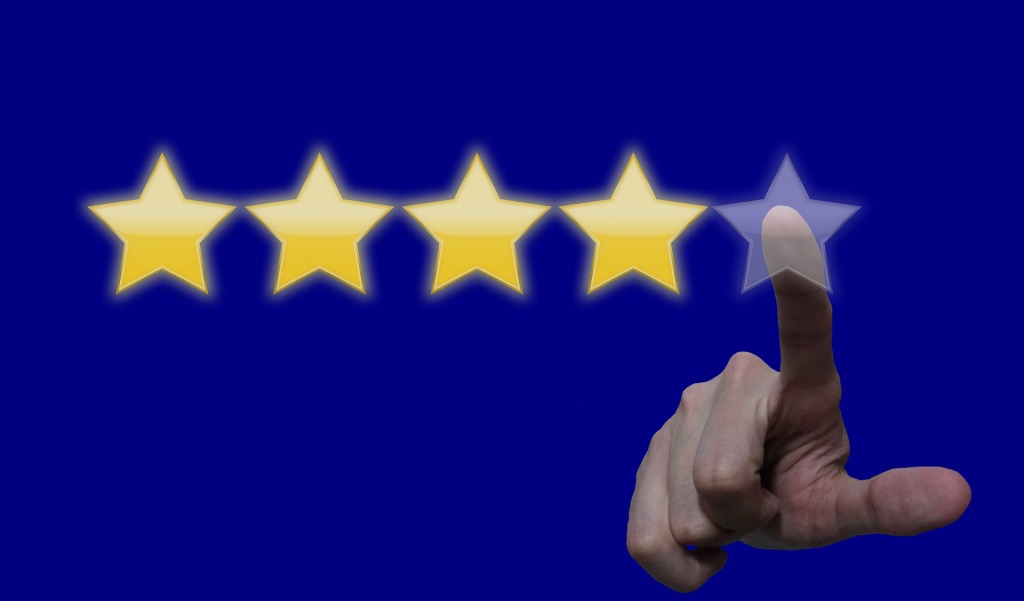
Current Standing on the Imposter Syndrome Scale
?/5
Like the Alaskan weather – it changes by the minute!